What do we mean by “conscious AI”?

The idea that AI can become conscious is worrying a lot of people – a conscious entity could resist control and even take control. Let’s break this up into sentience, consciousness and being self-aware.
Sentience is the capacity of a being to experience feelings and sensations. The word was first coined by philosophers in the 1630s for the concept of an ability to feel, derived from Latin sentiens (feeling), to distinguish it from the ability to think (reason). In modern Western philosophy, sentience is the ability to experience sensations. In different Asian religions, the word “sentience” has been used to translate a variety of concepts. In science fiction, the word “sentience” is sometimes used interchangeably with “sapience“, “self-awareness“, or “consciousness“.
Consciousness, at its simplest, is awareness of internal and external existence. However, its nature has led to millennia of analyses, explanations and debates by philosophers, theologians, linguists, and scientists. Opinions differ about what exactly needs to be studied or even considered consciousness.
In some explanations, it is synonymous with the mind, and at other times, an aspect of mind. In the past, it was one’s “inner life”, the world of introspection, of private thought, imagination and volition. Today, it often includes any kind of cognition, experience, feeling or perception. It may be awareness, awareness of awareness, or self-awareness either continuously changing or not. The disparate range of research, notions and speculations raises a curiosity about whether the right questions are being asked.
Bodily self-awareness in human development refers to one’s awareness of their body as a physical object, with physical properties, that can interact with other objects. Tests have shown that at the age of only a few months old, toddlers are already aware of the relationship between their own perception and visual information they receive. This is called first-person self-awareness.
At around 18 months old and later, children begin to develop reflective self-awareness, which is the next stage of bodily awareness and involves children recognising themselves in reflections, mirrors, and pictures. Children who have not obtained this stage of bodily self-awareness yet will tend to view reflections of themselves as other children and respond accordingly, as if they were looking at someone else face to face.
In contrast, those who have reached this level of awareness will recognise that they see themselves, for instance seeing dirt on their face in the reflection and then touching their own face to wipe it off.
Slightly after toddlers become reflectively self-aware, they begin to develop the ability to recognise their bodies as physical objects in time and space that interact and impact other objects. For instance, a toddler placed on a blanket, when asked to hand someone the blanket, will recognize that they need to get off it to be able to lift it. This is the final stage of body self-awareness and is called objective self-awareness.
What do we mean by AI? Artificial intelligence was developed around various aspects of human thought:
Reasoning, problem-solving
Early researchers developed algorithms that imitated step-by-step reasoning that humans use when they solve puzzles or make logical deductions. By the late 1980s and 1990s, methods were developed for dealing with uncertain or incomplete information, employing concepts from probability and economics. However, humans solve most of their problems using fast, intuitive judgements. Accurate and efficient reasoning is an unsolved problem.
Knowledge representation
An ontology represents knowledge as a set of concepts within a domain and the relationships between those concepts.
Knowledge representation and knowledge engineering allow AI programs to answer questions intelligently and make deductions about real-world facts. Formal knowledge representations are used in content-based indexing and retrieval, scene interpretation, clinical decision support, knowledge discovery (mining “interesting” and actionable inferences from large databases), and other areas.
A knowledge base is a body of knowledge represented in a form that can be used by a program. An ontology is the set of objects, relations, concepts, and properties used by domain of knowledge. The most general ontologies are called upper ontologies, which attempt to provide a foundation for all other knowledge and act as mediators between domain ontologies that cover specific knowledge about a particular domain (field of interest or area of concern).
Knowledge bases need to represent things such as: objects, properties, categories and relations between objects; situations, events, states and time; causes and effects; knowledge about knowledge (what we know about what other people know); default reasoning (things that humans assume are true until they are told differently and will remain true even when other facts are changing); and many other aspects and domains of knowledge.
Among the most difficult problems in knowledge representation are: the breadth of commonsense knowledge (the set of atomic facts that the average person knows) is enormous; the difficulty of knowledge acquisition and the sub-symbolic form of most commonsense knowledge (much of what people know is not represented as “facts” or “statements” that they could express verbally).
Learning
Machine learning is the study of programs that can improve their performance on a given task automatically. It has been a part of AI from the beginning.
There are several kinds of machine learning. Unsupervised learning analyses a stream of data and finds patterns and makes predictions without any other guidance. Supervised learning requires a human to label the input data first, and comes in two main varieties: classification (where the program must learn to predict what category the input belongs in) and regression (where the program must deduce a numeric function based on numeric input).
In reinforcement learning the agent is rewarded for good responses and punished for bad ones. The agent learns to choose responses that are classified as “good”. Transfer learning is when the knowledge gained from one problem is applied to a new problem. Deep learning uses artificial neural networks for all of these types of learning.
Computational learning theory can assess learners by computational complexity, by sample complexity (how much data is required), or by other notions of optimization.
Natural language processing
Natural language processing (NLP) allows programs to read, write and communicate in human languages such as English. Specific problems include speech recognition, speech synthesis, machine translation, information extraction, information retrieval and question answering.
Early work, based on Noam Chomsky‘s generative grammar, had difficulty with word-sense disambiguation unless restricted to small domains called “micro-worlds” (due to the common sense knowledge problem.
Modern deep learning techniques for NLP include word embedding (how often one word appears near another), transformers (which finds patterns in text), and others. In 2019, generative pre-trained transformer (or “GPT”) language models began to generate coherent text, and by 2023 these models were able to get human-level scores on the bar exam, SAT, GRE, and many other real-world applications.
Perception
Feature detection (edge detection) helps AI compose informative abstract structures out of raw data.
Machine perception is the ability to use input from sensors (such as cameras, microphones, wireless signals, active lidar, sonar, radar, and tactile sensors) to deduce aspects of the world. Computer vision is the ability to analyse visual input. The field includes speech recognition, image classification, facial recognition, object recognition, and robotic perception.
Social intelligence
Affective computing is an interdisciplinary umbrella that comprises systems that recognise, interpret, process or simulate human feeling, emotion and mood. For example, some virtual assistants are programmed to speak conversationally or even to banter humorously; it makes them appear more sensitive to the emotional dynamics of human interaction, or to otherwise facilitate human–computer interaction.
However, this tends to give naïve users an unrealistic conception of how intelligent existing computer agents actually are. Moderate successes related to affective computing include textual sentiment analysis and, more recently, multimodal sentiment analysis, wherein AI classifies the affects displayed by a videotaped subject.
General intelligence
A machine with artificial general intelligence should be able to solve a wide variety of problems with breadth and versatility similar to human intelligence.
From the above it appears logical to assume that a GPT, even with its tremendous store of data will not be able to achieve the degree of lateral thinking and fast intuitive judgemental abilities which make the human species able to achieve its dominant place in the world.
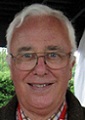
Alan Stevenson spent four years in the Royal Australian Navy; four years at a seminary in Brisbane and the rest of his life in computers as an operator, programmer and systems analyst. His interests include popular science, travel, philosophy and writing for Open Forum.